In this paper, we demonstrated techniques for generating accessories in the form of eyeglass frames that, when printed and worn,…
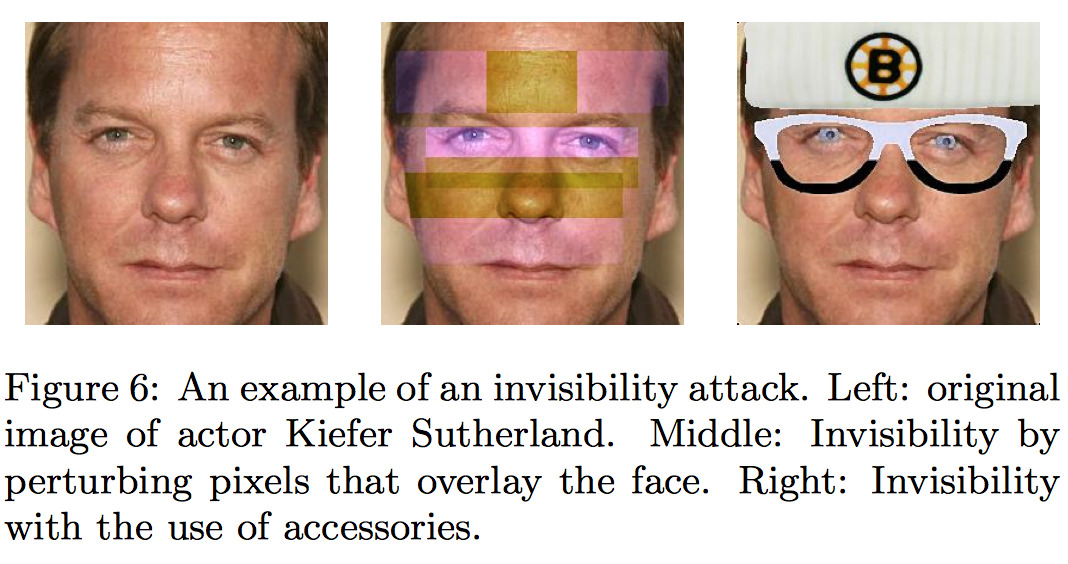
In this paper, we demonstrated techniques for generating accessories in the form of eyeglass frames that, when printed and worn, can effectively fool state-of-the-art face-recognition systems. Our research builds on recent research in fooling machine-learning classifiers by perturbing inputs in an adversarial way, but does so with attention to two novel goals: the perturbations must be physically realizable and inconspicuous. We showed that our eyeglass frames enabled subjects to both dodge recognition and to impersonate others. We believe that our demonstration of techniques to realize these goals through printed eyeglass frames is both novel and important, and should inform future deliberations on the extent to which ML can be trusted in adversarial settings. Finally, we extended our work in two additional directions, first, to so-called black-box FRSs that can be queried but for which the internals are not known, and, second, to defeat state-of-the-art face detection systems.